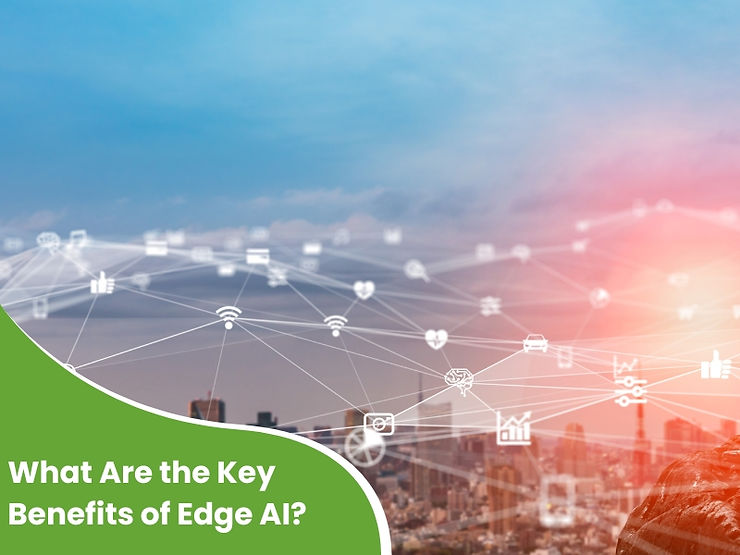
Edge AI is quickly becoming a game-changer in artificial intelligence. By processing data locally on devices rather than relying on cloud computing, Edge AI is reshaping industries and revolutionising how we interact with technology. But what exactly are the key benefits of this technology? This article will explore Edge AI's main benefits, real-world applications, and more.
What is Edge AI and How Does It Work?
Edge AI runs artificial intelligence models directly on devices at the edge of a network, including smartphones, wearables, cameras, and IoT sensors. Unlike traditional systems that send data to a central cloud for processing, Edge AI processes data locally, enabling real-time decision-making on the device itself.
By leveraging edge computing and AI, Edge AI enhances applications by reducing latency, ensuring better privacy, and reducing the need for continuous internet connectivity. It moves computational power closer to the data source, allowing smarter devices and more efficient processing. Lightweight AI models run directly on the device, making fast, real-time decisions without relying on cloud infrastructure. This makes Edge AI ideal for applications that require immediate action, even in areas with limited or no internet connectivity.
What Are the Main Benefits of Edge AI for Users?
Edge AI offers a range of benefits that can significantly improve the user experience across various industries. These include:
Faster Responses: Local data processing ensures that Edge AI can deliver real-time responses with minimal delay, making it ideal for time-sensitive applications like autonomous vehicles and industrial monitoring.
Improved Privacy: Data remains on the device, reducing the risks of exposing sensitive information to the cloud. Edge AI is a strong option for industries handling personal or confidential data.
Lower Bandwidth Usage: Edge AI minimises the need for constant data transmission, saving bandwidth and reducing internet costs, which is particularly beneficial for IoT devices and remote locations with limited connectivity.
Enhanced Reliability: Edge AI doesn’t rely on the cloud for processing, so devices can continue functioning even in areas with weak or no internet connection. This makes it ideal for use in remote locations or environments with unstable networks.
Cost Savings: By processing data locally, Edge AI reduces the need for expensive cloud storage and the associated costs of transmitting large volumes of data. It also helps conserve energy, making it more cost-effective for energy-intensive applications.
What Are the Real-World Applications of Localised AI?
Edge AI is already being utilised in various sectors, with applications across consumer technology, healthcare, manufacturing, and more. Some of the most notable use cases include:
Smart Speakers and Virtual Assistants: Devices like Amazon Alexa use Edge AI to detect wake words and process voice commands without sending data to the cloud. This results in quicker responses and improved functionality.
Health and Fitness Wearables: Smartwatches and fitness trackers use Edge AI to monitor real-time health data like step counts and heart rate. This allows users to receive immediate feedback without the need for cloud processing.
Manufacturing and Quality Control: Smart cameras with Edge AI can detect defects in production lines, ensuring faster and more accurate identification of issues such as packaging errors or faulty products.
Autonomous Vehicles: Autonomous cars rely heavily on Edge AI for obstacle detection, navigation, and decision-making tasks. With Edge AI, vehicles can process sensor data locally to make immediate, life-critical decisions.
Agriculture: In agriculture, Edge AI monitors crop health, soil conditions, and livestock movement. This provides farmers with real-time insights while reducing reliance on remote cloud services.
How Does Edge AI Contribute to Better AI Performance?
Edge AI accelerates AI processing by performing computations directly on the device, leading to faster decision-making and reduced latency. The following factors contribute to this:
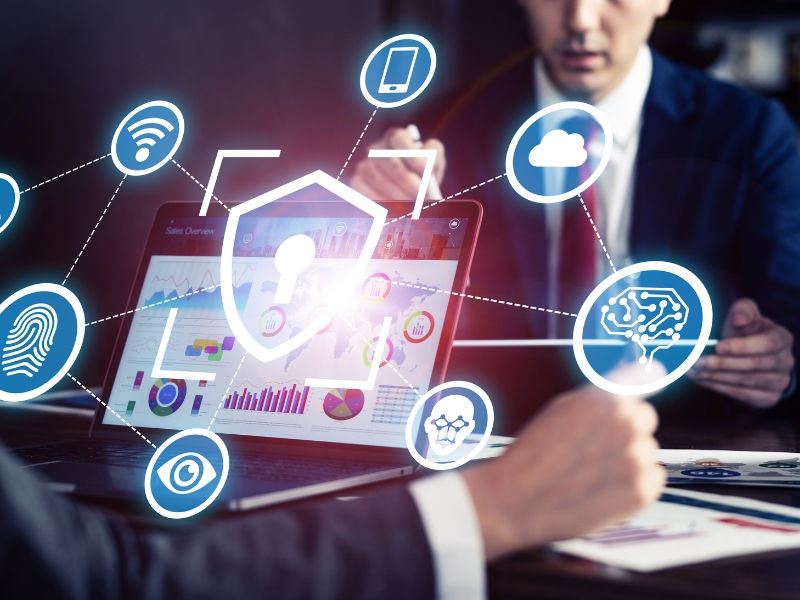
Reduced Latency: Edge AI drastically reduces latency by eliminating data needing to travel to the cloud. This is particularly critical for real-time applications like video surveillance or autonomous systems.
Optimised Data Processing: Edge AI devices are specifically designed to process data efficiently. This includes using model optimisation techniques such as quantisation and pruning to improve the speed and accuracy of AI algorithms.
Energy Efficiency: Edge AI devices are often designed with energy-saving technologies like low-power chips and processors. This reduces power consumption, making it possible to run AI applications for extended periods without draining the device's battery.
Increased Model Efficiency: Edge AI allows continuous, local learning and improvement. Devices can refine their models using feedback loops, enhancing their ability to make accurate predictions and decisions.
How Does This Technology Integrate with Other Advancements?
Edge AI is not just an isolated technology—it integrates seamlessly with other emerging advancements in the tech world. These include:
IoT: Edge AI and the Internet of Things (IoT) go hand in hand. IoT devices generate vast amounts of data, which Edge AI processes locally to enable real-time decision-making and improve system efficiency.
Distributed Computing: Understanding the role of distributed computing in artificial intelligence is key to enhancing the scalability and efficiency of Edge AI systems. This allows multiple devices to collaborate on processing tasks, distributing the workload for faster and more efficient AI operations.
5G Connectivity: The low latency and high bandwidth of 5G networks complement Edge AI by enabling faster data transfer and improving device responsiveness. This makes Edge AI even more powerful, especially for applications that require real-time performance.
Federated Learning: Edge AI benefits from federated learning, which allows devices to train machine learning models without sharing raw data. This enhances data privacy and security while continuously improving AI models.
Cloud Integration: While Edge AI works independently, it can also be integrated with cloud-based platforms for remote updates, model improvements, and data storage, creating a hybrid system that offers both local and cloud-based benefits. Businesses exploring such hybrid systems can also benefit from Splunk Observability Cloud, which provides enhanced monitoring, analysis, and troubleshooting capabilities.
What are the energy considerations for Edge AI?
Energy efficiency is key in Edge AI, especially as many devices run on batteries. Here are a few strategies to optimise energy consumption:
Model Optimisation: Reducing the size and complexity of AI models allows for faster processing and lower power consumption.
Low-Power Hardware: Edge AI devices use energy-efficient hardware, such as specialised processors (ASICs, TPUs), to balance performance and power consumption.
Dynamic Workloads: Devices can activate specific processes only when needed, saving energy during idle times. For example, a security camera may only activate when motion is detected.
Edge-Cloud Collaboration: For more complex tasks, Edge AI can offload specific processing to the cloud, balancing local and remote processing to optimise energy use.
What Are the Drawbacks of Edge AI?
While Edge AI offers many advantages, it is not without its challenges. Some of the main drawbacks include:
Limited Hardware: Edge devices often have limited computational power and memory, making running complex machine-learning models difficult.
Model Updates: Unlike cloud-based systems, updating AI models on multiple Edge devices can be more complicated and time-consuming.
Limited Accessibility: Edge AI may not provide the same level of universal access as cloud-based systems, especially for users in remote areas.
Security Concerns: Edge devices can be vulnerable to tampering and cyberattacks. Securing Edge AI systems requires additional measures, including encryption and regular software updates.
How Can You Overcome Security Challenges?
Security is a significant concern with Edge AI, mainly since sensitive data is often processed on local devices. Here are a few strategies to enhance security:

Encryption: Encryption: Encrypting data on the device ensures it remains unreadable, even if intercepted.
Secure Boot: Using secure boot processes prevents unauthorised firmware from being installed on devices.
Federated Learning: This method allows AI models to be trained across multiple devices without exposing raw data, helping to protect privacy.
Regular Software Updates: Keeping software up to date ensures that vulnerabilities are patched and the system is protected from cyberattacks.
Log Security: Understanding and implementing the importance of log security for threat detection helps prevent unauthorised access and strengthens overall security measures for Edge AI devices.
What Does the Future Hold for Edge AI?
The future of Edge AI is bright, with advancements in hardware, 5G, and other technologies pushing the boundaries of what is possible. We can expect:
More Autonomous Systems: As Edge AI evolves, we’ll see more autonomous systems, such as self-driving cars and drones, relying on real-time processing to make decisions without human intervention.
Improved Healthcare Solutions: Edge AI will be crucial in healthcare, offering real-time monitoring and personalised patient treatment options.
Smarter Cities: Integrating IoT and Edge AI will make cities smarter by improving traffic management, energy efficiency, and public safety.
Energy-Efficient AI Devices: Ongoing developments in low-power AI chips will make Edge AI even more energy-efficient, extending device battery life and reducing environmental impact.
Explore Edge AI with Ingeniq
Interested in learning more about Edge AI? Ingeniq offers comprehensive Splunk courses to help you understand how to harness the power of Edge AI for your business. To elevate your skills in cutting-edge technologies, learn more about Ingeniq and our specialised Splunk courses designed to empower your career. Start exploring today!
Frequently Asked Questions
What are the key differences between Edge AI and traditional AI?
Edge AI processes data locally on devices, reducing cloud reliance. At the same time, traditional AI uses cloud servers for data processing, often introducing latency.
Can Edge AI devices function without an internet connection?
Yes, Edge AI works without the internet as it processes data on the device, making it ideal for areas with poor connectivity.
How does Edge AI handle data privacy and security?
By performing computations directly on the device, Edge AI enhances data privacy. This approach minimises the amount of data transmitted to remote servers, keeping sensitive information within the local environment and bolstering overall security.
What kind of hardware is needed to support Edge AI applications?
Edge AI requires specialised hardware for local data processing, such as edge devices, sensors, and AI chips, such as NVIDIA Jetson or Intel Movidius.
Can Edge AI be integrated with existing AI systems?
Edge AI can complement cloud AI by handling real-time tasks and reducing cloud infrastructure load.
How does Edge AI reduce latency in real-time applications?
By processing data locally, Edge AI eliminates cloud communication delays, enabling faster decision-making in real-time applications.
What are the scalability challenges with Edge AI?
Scaling Edge AI involves managing many devices and hardware limitations and ensuring smooth updates and maintenance.
Can Edge AI improve the performance of consumer devices like smartphones and wearables?
Yes, Edge AI enhances performance by running AI models directly on devices, offering quicker responses and reducing cloud dependence.
How is Edge AI evolving in the field of machine learning?
Edge AI is evolving with better hardware and software, allowing more complex AI models to be deployed and improving real-time machine learning.
What impact will Edge AI have on cloud computing in the future?
Edge AI complements cloud computing by offloading real-time tasks to local devices, making cloud management more efficient.
コメント